Korea Machine Learning Ledger Orchestration for Drug Discovery
Supported by the Korean Ministry of Science and ICT and the Ministry of Health and Welfare (24.4 ~ 28.12, 5 years, $25 million USD)
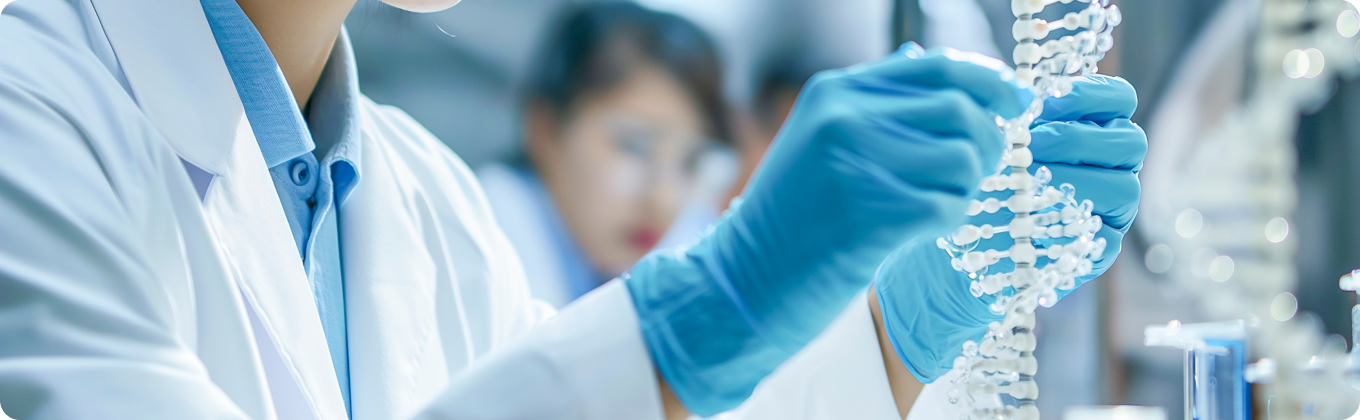
Project Objectives
- Establishing a federated learning-based AI drug discovery platform that enables the secure utilization of drug development-related data distributed across pharmaceutical companies, research institutes, universities, and hospitals, aiming to position the nation as a leader in AI-driven drug discovery.
Main Content
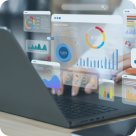
Platform
Establishment of a Federated Drug Discovery (FDD) platform for accelerated drug development
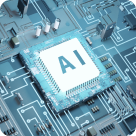
Development of a Federated ADMET Model (FAM) based on FDD Platform
Project Necessity
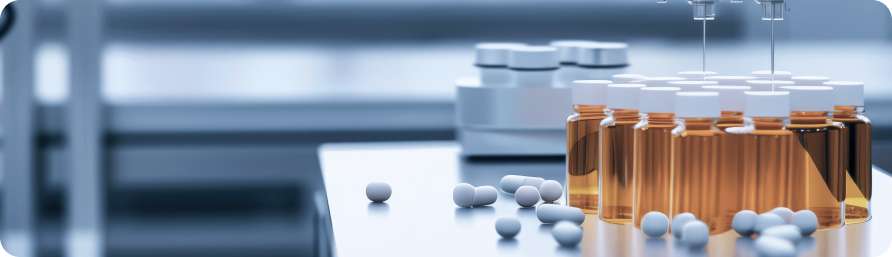
Importance of ADMET Prediction
- ADMET (Absorption, Distribution, Metabolism, Excretion, Toxicity) is the most crucial factor for the success of clinical trials and accounts for 22% of drug development R&D costs (NIH).
- It is difficult to guarantee the success of preclinical (in-vivo) and clinical trials based solely on in-vitro experimental results (limitations of stage-by-stage testing).
- Predicting ADMET clinical trial success requires using in-human clinical trial data for training, but sharing such comprehensive data across all stages is nearly impossible, making model implementation extremely challenging.
Sub Projects
Sub Project 1
Platform developer
(1Task)
Build a Federated Learning-based Drug Discovery (FDD) platform and operating the FAM solution.
- Define platform requirements, plan infrastructure development, and establish a data protection strategy
- Verify safety through the development and operation of platform performance, usability, and security features
- Improve reward mechanisms for each user, and develop plans for platform expansion and commercialization
Sub Project 2
Data owners
(20Task)
Providing data from pharmaceutical companies, universities, hospitals, and research institutions and utilizing the FAM model.
- Define AI solution tasks necessary for ADMET/PK prediction, assess data, and design data supply plans
- Build data for training the base model and developing preprocessing tools
- Design new data supply methods, monitor solution performance, and provide feedback on usage outcomes
Sub Project 3
AI model providers
(15Task)
Development of FAM solutions and application models (selecting 5 projects each year for three years).
- Analyze solution requirements, design preprocessing tools, and develop AI models
- Improve performance in cases of data imbalance, missing values, and fine-tuning application
- Compare performance with existing ADMET/PK prediction models by task, and study commercialization strategies for the solution
FAM
- FAM is Federated ADMET Model
- A model that predicts ADMET and clinical trial PK (pharmacokinetic) parameters as final endpoints by integrating in-vitro, in-vivo, and clinical trial (in-human) data.
- Not just a one-time solution, but a model that continuously and automatically enhances its performance with new data.
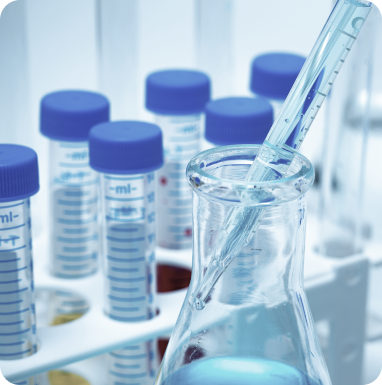
Expected Outcomes
- Establishing a Data-Driven Open Innovation
Ecosystem
- Establishing an open innovation ecosystem through federated learning, facilitating collaboration among competitive institutions using their own data.
- AI solution development companies verify and deploy performance in real time on a platform, rather than relying on one-to-one agreements with data-holding institutions.
- Becoming a Leading Country in AI-Based
Drug Development
- By securing a full-cycle (in vitro, in vivo, in human) integrated prediction model for ADMET/PK, we can reduce Korea’s drug development R&D costs and time, while increasing success rates
- Enables the utilization of various types of data held by domestic and international institutions
- Expanding the Data Utilization
Ecosystem
- Significantly reduces the procedures for data production, transfer, processing, and storage needed for AI model training, with each institution managing its original data, thereby drastically reducing data management costs
- In federated learning, there is fundamentally no security threat from the physical transfer of data, enabling “shared utilization” of data among institutions, which is expected to greatly increase its application in the healthcare field